About
I am a PostDoc with the group of Prof. Lyudmila Grigoryeva at the Mathematics and Statistics Division, School of Economics and Political Science, University of St. Gallen thanks to the Great Minds Postdoctoral Fellowship program.
I completed my PhD in Economics at the Graduate School of Economic and Social Sciences, University of Mannheim.
My main research interests are time series econometrics, nonparametric statistics and statistical/machine learning, with a focus on macroeconomic applications.
Publications
- Ridge Regularized Estimation of VAR Models for Inference
Abstract
Ridge regression is a popular regularization method that has wide applicability, as many regression problems can be cast in this form. However, ridge is only seldom applied in the estimation of vector autoregressive models, even though it naturally arises in Bayesian time series modeling. In this work, ridge regression is studied in the context of process estimation and inference of VARs. The effects of shrinkage are analyzed and asymptotic theory is derived enabling inference. Frequentist and Bayesian ridge approaches are compared. Finally, the estimation of impulse response functions is evaluated with Monte Carlo simulations and ridge regression is compared with a number of similar and competing methods.
- Memory of Recurrent Networks: Do We Compute It Right?
Abstract
Numerical evaluations of the memory capacity (MC) of recurrent neural networks reported in the literature often contradict well-established theoretical bounds. In this paper, we study the case of linear echo state networks, for which the total memory capacity has been proven to be equal to the rank of the corresponding Kalman controllability matrix. We shed light on various reasons for the inaccurate numerical estimations of the memory, and we show that these issues, often overlooked in the recent literature, are of an exclusively numerical nature. More explicitly, we prove that when the Krylov structure of the linear MC is ignored, a gap between the theoretical MC and its empirical counterpart is introduced. As a solution, we develop robust numerical approaches by exploiting a result of MC neutrality with respect to the input mask matrix. Simulations show that the memory curves that are recovered using the proposed methods fully agree with the theory.
- Reservoir Computing for Macroeconomic Forecasting with Mixed Frequency Data
Abstract
Macroeconomic forecasting has recently started embracing techniques that can deal with large-scale datasets and series with unequal release periods. The aim is to exploit the information contained in heterogeneous data sampled at different frequencies to improve forecasting exercises. Currently, MIxed-DAta Sampling (MIDAS) and Dynamic Factor Models (DFM) are the two main state-of-the-art approaches that allow modeling series with non-homogeneous frequencies. We introduce a new framework called the Multi-Frequency Echo State Network (MFESN), which originates from a relatively novel machine learning paradigm called reservoir computing (RC). Echo State Networks are recurrent neural networks with random weights and trainable readout. They are formulated as nonlinear state-space systems with random state coefficients where only the observation map is subject to estimation. This feature makes the estimation of MFESNs considerably more efficient than DFMs. In addition, the MFESN modeling framework allows to incorporate many series, as opposed to MIDAS models, which are prone to the curse of dimensionality. Our discussion encompasses hyperparameter tuning, penalization, and nonlinear multistep forecast computation. In passing, a new DFM aggregation scheme with Almon exponential structure is also presented, bridging MIDAS and dynamic factor models. All methods are compared in extensive multistep forecasting exercises targeting US GDP growth. We find that our ESN models achieve comparable or better performance than MIDAS and DFMs at a much lower computational cost.
Working Papers
- Memory Capacity of Nonlinear Recurrent Networks: Is it Informative?
Abstract
The total memory capacity (MC) of linear recurrent neural networks (RNNs) has been proven to be equal to the rank of the corresponding Kalman controllability matrix, and it is almost surely maximal for connectivity and input weight matrices drawn from regular distributions. This fact questions the usefulness of this metric in distinguishing the performance of linear RNNs in the processing of stochastic signals. This note shows that the MC of random nonlinear RNNs yields arbitrary values within established upper and lower bounds depending just on the input process scale. This confirms that the existing definition of MC in linear and nonlinear cases has no practical value.
- Impulse Response Analysis of Structural Nonlinear Time Series Models
[ArXiv] — Feb 2025 update!
Abstract
This paper proposes a semiparametric sieve approach to estimate impulse response functions of nonlinear time series within a general class of structural autoregressive models. We prove that a two-step procedure can flexibly accommodate nonlinear specifications while avoiding the need to choose fixed parametric forms. Sieve impulse responses are proven to be consistent by deriving uniform estimation guarantees, and an iterative algorithm makes it straightforward to compute them in practice. With simulations, we show that the proposed semiparametric approach proves effective against misspecification while suffering only from minor efficiency losses. In a US monetary policy application, we find that the pointwise sieve GDP response associated with an interest rate increase is larger than that of a linear model. Finally, in an analysis of interest rate uncertainty shocks, sieve responses imply more substantial contractionary effects both on production and inflation.
Work in Progress
- Theory of Nonparametric Regression with Random-Weights Neural Networks
- Multi-Frequency Economic Forecasting with Reservoir Ensembles
- Shapley Value Analysis of Reservoir Systems
- On the Limiting Distribution of Sieve VAR(∞) Estimators in Small Samples
Teaching
-
University of Mannheim:
-
(TA) Advanced Econometrics I - Winter Semester 2021, 2022, 2023
-
(TA) Advanced Macroeconomics III - Summer Semester 2021
-
(TA) Mathematics for Economists - Winter Semester 2019, 2020
-
Projects
- Implementation of Multi-Frequency ESN Models at the KOF Nowcasting Lab, ETH Zürich - February 2023
Many thanks to Maurizio Daniele, Heiner Mikosch and KOF for the opportunity and hospitality!
Update: Since December 2024, MFESN models have been permanently included in the suite of forecasting models at the Nowcasting Lab. For more information, read the original blog post!
-
GPU-Accelerated Value Function Iteration in Julia - JuliaCon 2018
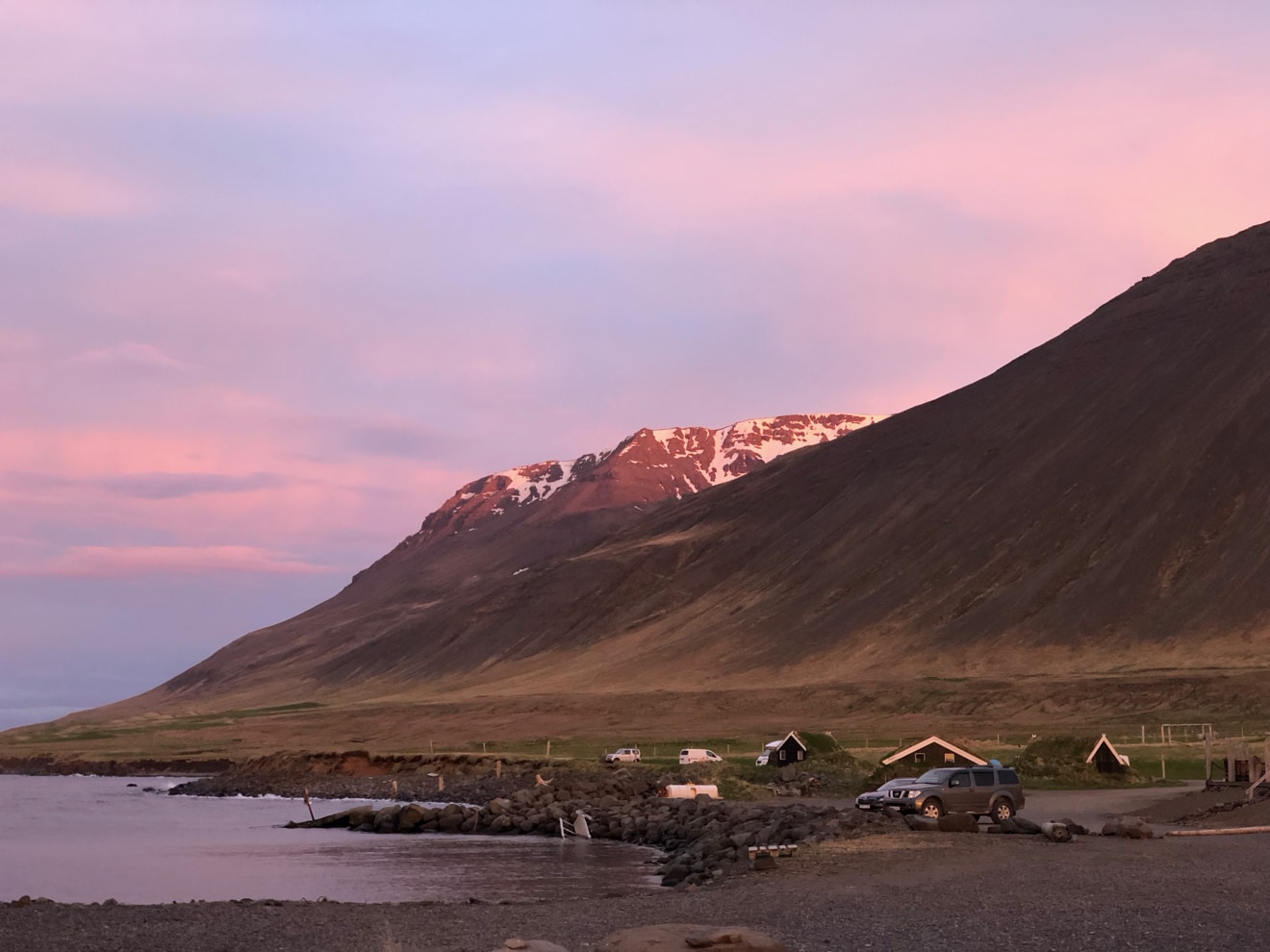